The skills required for a Data Analyst include technical proficiencies, domain-specific knowledge, and soft skills crucial for succeeding in the data analytics job market. Let us examine these in detail below.
Technical Skills
Any duty associated with data analysis requires well-developed skills or technical capabilities. So, building a career in Data Analysis means building various sets of technical skills, including programming skills. Below, we have compiled and briefly explained major Data Analyst technical skills for you to explore.
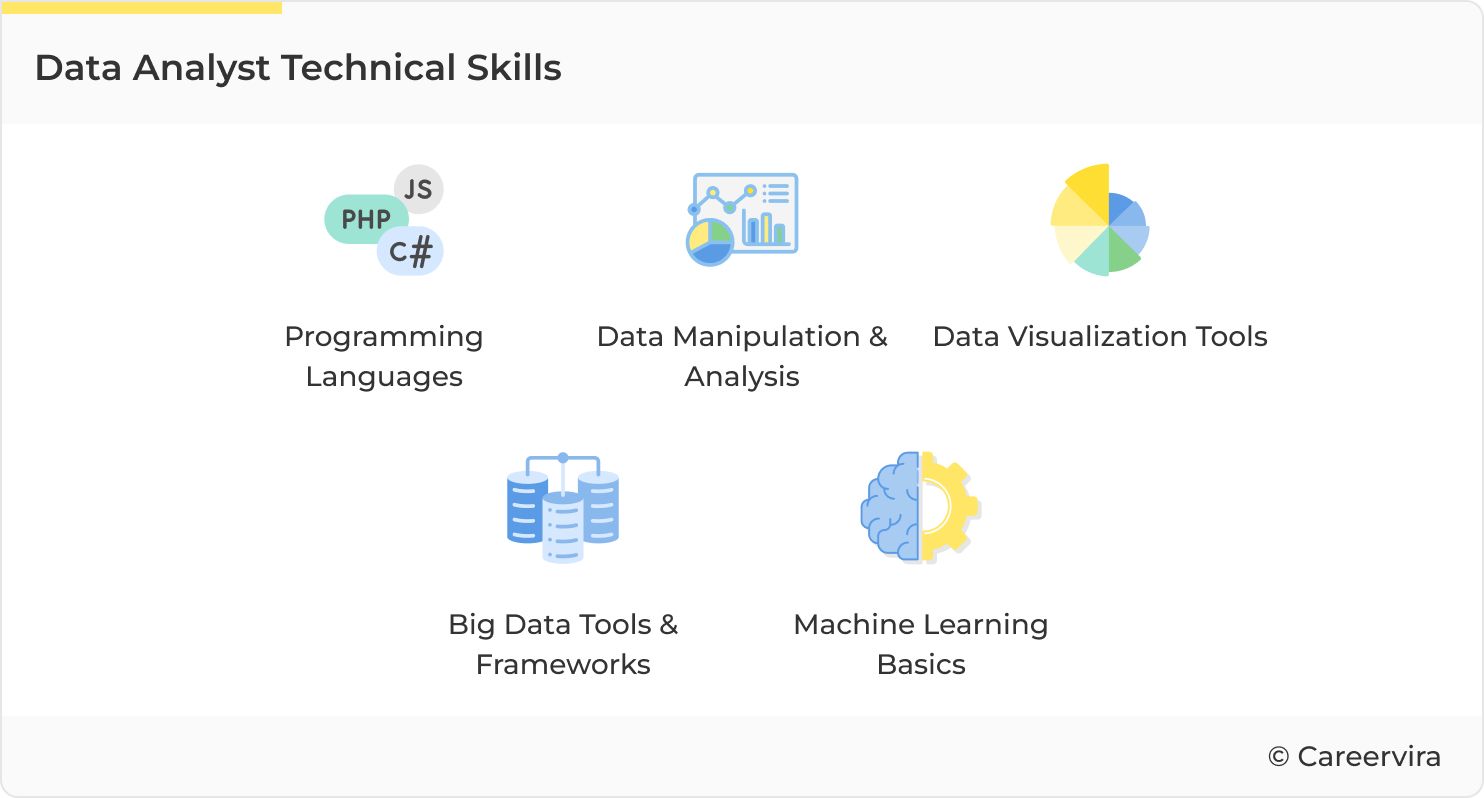
- Python Programming Language
Python has become the first choice for data analysts due to its simplicity, versatility, and extensive library support for data manipulation and analysis. The language is easy to learn and has a strong community, making it an excellent choice for beginners and experienced analysts alike. There are various uses of Python for Data Analysts, so getting your basics in Python strong will help you in the long run.
Data analysts use Python for:- Data Manipulation
Python's extensive libraries, such as Pandas and NumPy, allow data analysts to efficiently clean, preprocess, and transform data to make it suitable for analysis.
- Data Visualization
Data analysts can create insightful visualizations using Python libraries like Matplotlib, Seaborn, and Plotly, which help communicate findings and trends to stakeholders.
- Statistical Analysis
Python offers libraries like SciPy and StatsModels, enabling data analysts to perform various statistical tests, modeling, and hypothesis testing.
- Machine Learning
Python's Scikit-learn library provides a wide range of algorithms and tools to train, evaluate, and fine-tune models for data analysts working with machine learning models.
- Data Manipulation
- R Programming
R is a popular statistical computing and graphics language used in academia and research. It offers a rich ecosystem of packages and tools specifically designed for data analysis, making it an essential skill for data analysts working with complex statistical models.
Data analysts use R for:- Data Manipulation
R's dplyr package simplifies data cleaning and preprocessing tasks, making it easier for data analysts to filter, sort, and aggregate data.
- Data Visualization
R's ggplot2 library allows data analysts to create elegant and customizable visualizations. It also helps in the exploration and communication of data insights.
- Statistical Analysis
R's core functionality and numerous packages, such as LM and GLM, provide data analysts with the tools to perform various statistical tests and modeling techniques.
- Machine Learning
Data analysts can leverage R's caret package to train, evaluate, and optimize machine learning models, making it easier to work with complex algorithms.
- Data Manipulation
- SQL
SQL is a domain-specific language used for managing and querying relational databases. As data analysts often need to retrieve and manipulate data stored in databases, proficiency in SQL is crucial for efficiently extracting and filtering relevant information.
Data analysts use SQL for:- Query Databases
SQL enables data analysts to extract relevant information from databases by writing SELECT queries that filter, sort, and aggregate data.
- Data Manipulation
Data analysts can use SQL to clean and preprocess data, using commands like UPDATE, INSERT, and DELETE to modify database records.
- Data Aggregation
SQL's aggregation functions, such as SUM, COUNT, AVG, MIN, and MAX, allow data analysts to perform calculations on data groups, enabling the identification of patterns and trends.
- Joining Tables
SQL's JOIN operations enable data analysts to combine information from multiple tables in a database, providing a more comprehensive view of the data and facilitating deeper analysis.
- Query Databases
- Data Manipulation and Analysis
These are critical components of the data analyst's skill set, as they involve transforming raw data into meaningful insights that can drive informed decision-making. Data analysts must be proficient in data manipulation techniques, such as cleaning, filtering, aggregating, and transforming data, to ensure its accuracy and reliability before performing any analysis.
Data cleaning is essential in data manipulation, as raw data often contains inconsistencies, errors, or missing values. Data analysts must identify and address these issues to maintain the integrity of their analyses. Techniques such as imputing missing values, removing duplicates, correcting data entry errors, and standardizing data formats are employed to ensure the data is clean and ready for analysis.
Data analysts can proceed to the analysis stage once the data is properly cleaned and preprocessed. This involves using various statistical methods, algorithms, and data visualization techniques to uncover patterns, trends, and relationships within the data. Data analysts may employ descriptive statistics to summarize the data, inferential statistics to make predictions or identify correlations, and Exploratory Data Analysis (EDA) to generate hypotheses and identify potential areas of interest for further investigation.
Familiarity with tools and libraries - Pandas (Python)
- dplyr (R)
- NumPy (Python)
are essential for efficient data manipulation and wrangling.
Ultimately, data manipulation and analysis aim to transform raw data into actionable insights that can be used to drive strategic decision-making.
By mastering these skills, data analysts can help organizations understand their customers, optimize their operations, and make more informed decisions. This will ultimately improve the company’s performance and enable it to compete effectively against its rivals.
- Data Visualization Tools
Data visualization tools are essential for data analysts, as they enable the transformation of complex data into clear and understandable visual representations. By using these tools, data analysts can communicate insights and findings more effectively to stakeholders, making it easier for decision-makers to grasp the significance of the data and make informed decisions based on the presented information.
There is a wide array of data visualization tools in the market, each with unique features and capabilities. Some popular tools include Tableau, Power BI, and QlikView, which offer user-friendly drag-and-drop interfaces and various visualization options such as bar charts, line charts, pie charts, and heat maps.
These tools also enable data analysts to create interactive dashboards for stakeholders to explore and gain deeper insights by filtering, sorting, and drilling the data. In addition to specialized tools like Tableau and Power BI, programming languages like Python and R provide libraries that enable data analysts to create custom visualizations.
- Big Data Tools and Frameworks
Due to the continual growth of volume, velocity, and variety, traditional data processing and storage methods struggle to continue with the increasing scale and complexity. This has led to the development of big data tools and frameworks that enable data analysts to efficiently process, store, and analyze vast amounts of data.
Big data tools are crucial in helping organizations derive valuable insights from the massive amounts of data they collect, ultimately leading to better decision-making and improved performance.
One of the most widely used big data frameworks is Apache Hadoop, an open-source platform for distributed storage and large dataset processing. Hadoop's primary components are the Hadoop Distributed File System (HDFS), which provides scalable and fault-tolerant storage, and the MapReduce programming model, which allows parallel processing of large datasets.
Data analysts must have big data skills to work with large-scale datasets and perform complex analyses effectively. By mastering these technologies, data analysts can help organizations discover the full potential of their data, enabling them to uncover hidden patterns, identify trends, and make data-driven decisions that improve performance.
- Machine Learning Basics
While a basic understanding of machine learning concepts and techniques can provide data analysts with a competitive edge, familiarity with tools like scikit-learn (Python) and TensorFlow can help analysts build predictive models and uncover hidden patterns in the data.
These are the most in-demand data science skills based on employer requirements, industry trends, and expert opinions. They represent a combination of essential technical skills, domain-specific knowledge, and cutting-edge technologies that are shaping the future of data analysis.
By mastering these skills, data analysts can increase their marketability, stay ahead of the competition, and contribute to solving complex business problems.
Soft Skills
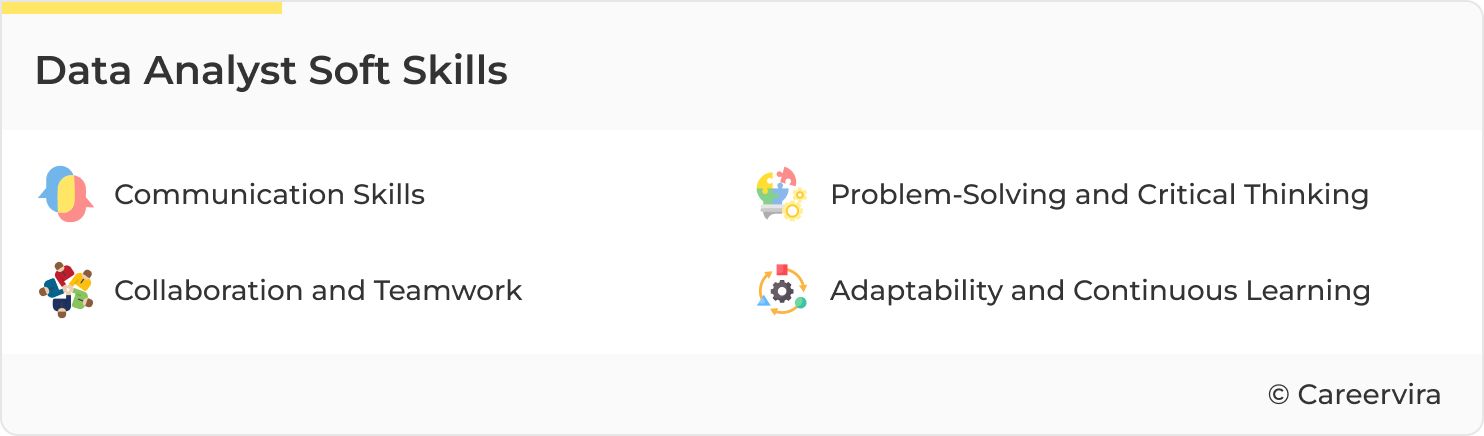
A comprehensive skill set makes you more attractive to potential employers and enables you to tackle various data analysis tasks, ultimately increasing your value as a professional. Here are the top Data Analyst soft skills that make your job easier.
- Communication Skills
- Presenting Findings To Non-Technical Stakeholders
Data analysts must learn to effectively communicate their findings to colleagues and decision-makers needing a technical background. This includes simplifying complex concepts, using relatable analogies, and creating visual aids to convey the insights clearly.
- Strong Presentation Skills
This is essential for ensuring that the organization understands and acts upon the value of the analysis.
- Creating Clear and Concise Reports
Data analysts must prepare well-structured, easy-to-understand reports summarizing their analyses and recommendations. These reports should be tailored to the audience, focusing on clarity and brevity, and must have visual appeal. Effective written communication is crucial for conveying insights and ensuring they have the desired impact.
- Presenting Findings To Non-Technical Stakeholders
- Problem-solving and Critical Thinking
Data analysts often face complex, ambiguous problems that require creative and analytical thinking. They must be able to break down these problems into manageable parts and develop logical, data-driven solutions. Critical thinking skills enable analysts to question assumptions, evaluate the quality of data, and identify potential biases that may affect their conclusions.
- Collaboration and Teamwork
Data analysts and Data scientists often work together. Data Analysts also often collaborate with cross-functional teams, including domain experts. Effective teamwork and collaboration skills are thus essential for fostering a productive work environment, sharing knowledge, and ensuring that the analysis is aligned with the organization's goals and priorities. Data Analysts often prefer a Data Scientist career path once they are experienced and consider a career progression.
- Adaptability and Continuous Learning
The data science field is constantly evolving with new tools, techniques, and technologies. Data analysts must be adaptable and willing to learn continuously. They must also stay up-to-date with industry trends and maintain their competitive edge.
The skills required for a Data Analyst include technical proficiencies, domain-specific knowledge, and soft skills crucial for succeeding in the data analytics job market. Let us examine these in detail below.
Technical Skills
Any duty associated with data analysis requires well-developed skills or technical capabilities. So, building a career in Data Analysis means building various sets of technical skills, including programming skills. Below, we have compiled and briefly explained major Data Analyst technical skills for you to explore.
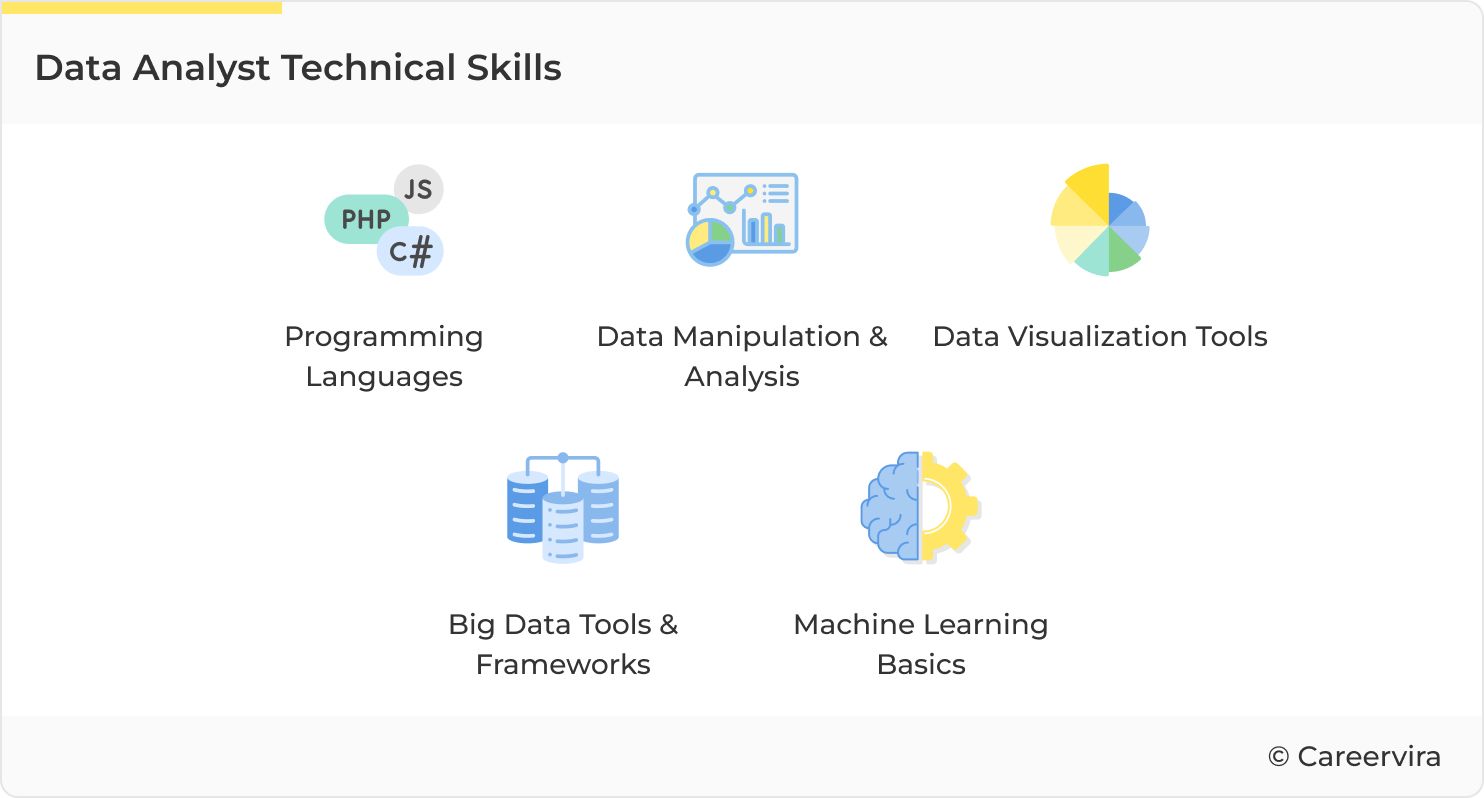
- Python Programming Language
Python has become the first choice for data analysts due to its simplicity, versatility, and extensive library support for data manipulation and analysis. The language is easy to learn and has a strong community, making it an excellent choice for beginners and experienced analysts alike. There are various uses of Python for Data Analysts, so getting your basics in Python strong will help you in the long run.
Data analysts use Python for:- Data Manipulation
Python's extensive libraries, such as Pandas and NumPy, allow data analysts to efficiently clean, preprocess, and transform data to make it suitable for analysis.
- Data Visualization
Data analysts can create insightful visualizations using Python libraries like Matplotlib, Seaborn, and Plotly, which help communicate findings and trends to stakeholders.
- Statistical Analysis
Python offers libraries like SciPy and StatsModels, enabling data analysts to perform various statistical tests, modeling, and hypothesis testing.
- Machine Learning
Python's Scikit-learn library provides a wide range of algorithms and tools to train, evaluate, and fine-tune models for data analysts working with machine learning models.
- Data Manipulation
- R Programming
R is a popular statistical computing and graphics language used in academia and research. It offers a rich ecosystem of packages and tools specifically designed for data analysis, making it an essential skill for data analysts working with complex statistical models.
Data analysts use R for:- Data Manipulation
R's dplyr package simplifies data cleaning and preprocessing tasks, making it easier for data analysts to filter, sort, and aggregate data.
- Data Visualization
R's ggplot2 library allows data analysts to create elegant and customizable visualizations. It also helps in the exploration and communication of data insights.
- Statistical Analysis
R's core functionality and numerous packages, such as LM and GLM, provide data analysts with the tools to perform various statistical tests and modeling techniques.
- Machine Learning
Data analysts can leverage R's caret package to train, evaluate, and optimize machine learning models, making it easier to work with complex algorithms.
- Data Manipulation
- SQL
SQL is a domain-specific language used for managing and querying relational databases. As data analysts often need to retrieve and manipulate data stored in databases, proficiency in SQL is crucial for efficiently extracting and filtering relevant information.
Data analysts use SQL for:- Query Databases
SQL enables data analysts to extract relevant information from databases by writing SELECT queries that filter, sort, and aggregate data.
- Data Manipulation
Data analysts can use SQL to clean and preprocess data, using commands like UPDATE, INSERT, and DELETE to modify database records.
- Data Aggregation
SQL's aggregation functions, such as SUM, COUNT, AVG, MIN, and MAX, allow data analysts to perform calculations on data groups, enabling the identification of patterns and trends.
- Joining Tables
SQL's JOIN operations enable data analysts to combine information from multiple tables in a database, providing a more comprehensive view of the data and facilitating deeper analysis.
- Query Databases
- Data Manipulation and Analysis
These are critical components of the data analyst's skill set, as they involve transforming raw data into meaningful insights that can drive informed decision-making. Data analysts must be proficient in data manipulation techniques, such as cleaning, filtering, aggregating, and transforming data, to ensure its accuracy and reliability before performing any analysis.
Data cleaning is essential in data manipulation, as raw data often contains inconsistencies, errors, or missing values. Data analysts must identify and address these issues to maintain the integrity of their analyses. Techniques such as imputing missing values, removing duplicates, correcting data entry errors, and standardizing data formats are employed to ensure the data is clean and ready for analysis.
Data analysts can proceed to the analysis stage once the data is properly cleaned and preprocessed. This involves using various statistical methods, algorithms, and data visualization techniques to uncover patterns, trends, and relationships within the data. Data analysts may employ descriptive statistics to summarize the data, inferential statistics to make predictions or identify correlations, and Exploratory Data Analysis (EDA) to generate hypotheses and identify potential areas of interest for further investigation.
Familiarity with tools and libraries - Pandas (Python)
- dplyr (R)
- NumPy (Python)
are essential for efficient data manipulation and wrangling.
Ultimately, data manipulation and analysis aim to transform raw data into actionable insights that can be used to drive strategic decision-making.
By mastering these skills, data analysts can help organizations understand their customers, optimize their operations, and make more informed decisions. This will ultimately improve the company’s performance and enable it to compete effectively against its rivals.
- Data Visualization Tools
Data visualization tools are essential for data analysts, as they enable the transformation of complex data into clear and understandable visual representations. By using these tools, data analysts can communicate insights and findings more effectively to stakeholders, making it easier for decision-makers to grasp the significance of the data and make informed decisions based on the presented information.
There is a wide array of data visualization tools in the market, each with unique features and capabilities. Some popular tools include Tableau, Power BI, and QlikView, which offer user-friendly drag-and-drop interfaces and various visualization options such as bar charts, line charts, pie charts, and heat maps.
These tools also enable data analysts to create interactive dashboards for stakeholders to explore and gain deeper insights by filtering, sorting, and drilling the data. In addition to specialized tools like Tableau and Power BI, programming languages like Python and R provide libraries that enable data analysts to create custom visualizations.
- Big Data Tools and Frameworks
Due to the continual growth of volume, velocity, and variety, traditional data processing and storage methods struggle to continue with the increasing scale and complexity. This has led to the development of big data tools and frameworks that enable data analysts to efficiently process, store, and analyze vast amounts of data.
Big data tools are crucial in helping organizations derive valuable insights from the massive amounts of data they collect, ultimately leading to better decision-making and improved performance.
One of the most widely used big data frameworks is Apache Hadoop, an open-source platform for distributed storage and large dataset processing. Hadoop's primary components are the Hadoop Distributed File System (HDFS), which provides scalable and fault-tolerant storage, and the MapReduce programming model, which allows parallel processing of large datasets.
Data analysts must have big data skills to work with large-scale datasets and perform complex analyses effectively. By mastering these technologies, data analysts can help organizations discover the full potential of their data, enabling them to uncover hidden patterns, identify trends, and make data-driven decisions that improve performance.
- Machine Learning Basics
While a basic understanding of machine learning concepts and techniques can provide data analysts with a competitive edge, familiarity with tools like scikit-learn (Python) and TensorFlow can help analysts build predictive models and uncover hidden patterns in the data.
These are the most in-demand data science skills based on employer requirements, industry trends, and expert opinions. They represent a combination of essential technical skills, domain-specific knowledge, and cutting-edge technologies that are shaping the future of data analysis.
By mastering these skills, data analysts can increase their marketability, stay ahead of the competition, and contribute to solving complex business problems.
Soft Skills
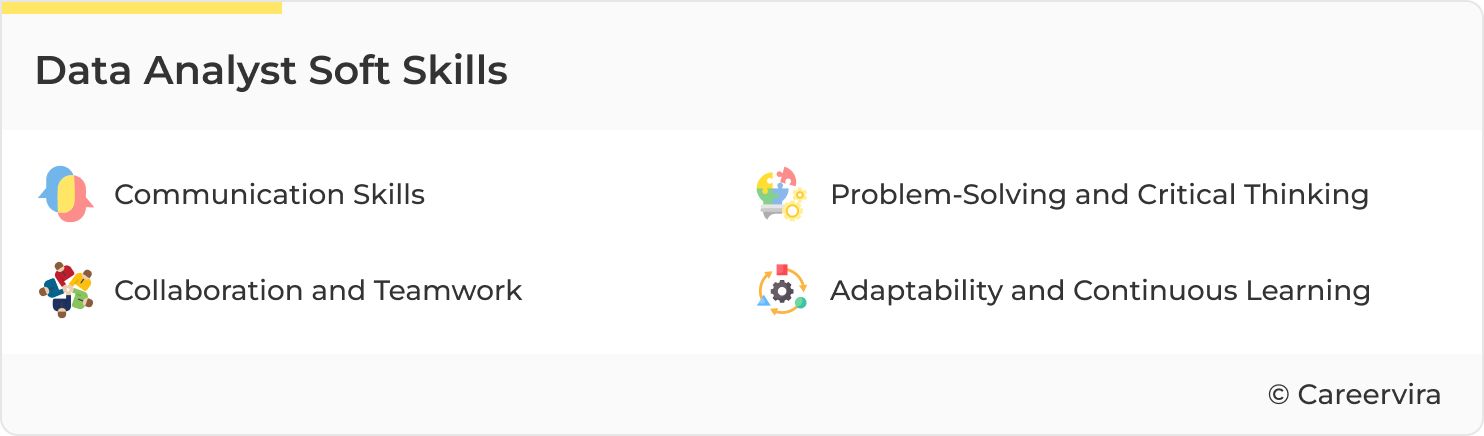
A comprehensive skill set makes you more attractive to potential employers and enables you to tackle various data analysis tasks, ultimately increasing your value as a professional. Here are the top Data Analyst soft skills that make your job easier.
- Communication Skills
- Presenting Findings To Non-Technical Stakeholders
Data analysts must learn to effectively communicate their findings to colleagues and decision-makers needing a technical background. This includes simplifying complex concepts, using relatable analogies, and creating visual aids to convey the insights clearly.
- Strong Presentation Skills
This is essential for ensuring that the organization understands and acts upon the value of the analysis.
- Creating Clear and Concise Reports
Data analysts must prepare well-structured, easy-to-understand reports summarizing their analyses and recommendations. These reports should be tailored to the audience, focusing on clarity and brevity, and must have visual appeal. Effective written communication is crucial for conveying insights and ensuring they have the desired impact.
- Presenting Findings To Non-Technical Stakeholders
- Problem-solving and Critical Thinking
Data analysts often face complex, ambiguous problems that require creative and analytical thinking. They must be able to break down these problems into manageable parts and develop logical, data-driven solutions. Critical thinking skills enable analysts to question assumptions, evaluate the quality of data, and identify potential biases that may affect their conclusions.
- Collaboration and Teamwork
Data analysts and Data scientists often work together. Data Analysts also often collaborate with cross-functional teams, including domain experts. Effective teamwork and collaboration skills are thus essential for fostering a productive work environment, sharing knowledge, and ensuring that the analysis is aligned with the organization's goals and priorities. Data Analysts often prefer a Data Scientist career path once they are experienced and consider a career progression.
- Adaptability and Continuous Learning
The data science field is constantly evolving with new tools, techniques, and technologies. Data analysts must be adaptable and willing to learn continuously. They must also stay up-to-date with industry trends and maintain their competitive edge.